Unified Scene Text Detection のインストールとテスト実行(テキスト検出)(Python,TensorFlow を使用)(Windows 上)
Unified Scene Text Detection をインストールし,テキスト検出(text detection)を行う.
【目次】
Unified Scene Text Detection
Unified Scene Text Detection は,テキスト検出(text detection),ドキュメントのレイアウト解析(layout analysis)を統合することにより,精度の改善を行っている. 2022年発表.
【文献】
Towards End-to-End Unified Scene Text Detection and Layout Analysis, Long, Shangbang and Qin, Siyang and Panteleev, Dmitry and Bissacco, Alessandro and Fujii, Yasuhisa and Raptis, Michalis, Proceedings of the IEEE/CVF Conference on Computer Vision and Pattern Recognition, 2022.
https://arxiv.org/abs/2203.15143
【関連する外部ページ】
- Unified Scene Text Detection の GitHub の公式ページ: https://github.com/tensorflow/models/tree/master/official/projects/unified_detector
- Paper with Code のページ: https://paperswithcode.com/paper/towards-end-to-end-unified-scene-text
前準備
Python 3.10,Git,7-Zip のインストール(Windows 上)
Pythonは,プログラミング言語の1つ. Gitは,分散型のバージョン管理システム. 7-Zipは,ファイル圧縮・展開(解凍)ツール.
【手順】
- Windows で,管理者権限でコマンドプロンプトを起動(手順:Windowsキーまたはスタートメニュー >
cmd
と入力 > 右クリック > 「管理者として実行」)。次のコマンドを実行
次のコマンドは,Python ランチャーとPython 3.10とGitと7-Zipをインストールし,Gitと7-Zipにパスを通すものである.
次のコマンドでインストールされるGitは 「git for Windows」と呼ばれるものであり, Git,MinGW などから構成されている.
winget install --scope machine Python.Launcher winget install --scope machine Python.Python.3.10 winget install --scope machine Git.Git powershell -command "$oldpath = [System.Environment]::GetEnvironmentVariable(\"Path\", \"Machine\"); $oldpath += \";c:\Program Files\Git\cmd\"; [System.Environment]::SetEnvironmentVariable(\"Path\", $oldpath, \"Machine\")" winget install --scope machine 7zip.7zip powershell -command "$oldpath = [System.Environment]::GetEnvironmentVariable(\"Path\", \"Machine\"); $oldpath += \";c:\Program Files\7-Zip\"; [System.Environment]::SetEnvironmentVariable(\"Path\", $oldpath, \"Machine\")"
【関連する外部ページ】
- Python の公式ページ: https://www.python.org/
- Git の公式ページ: https://git-scm.com/
- 7-Zip の公式ページ: https://7-zip.opensource.jp/
【サイト内の関連ページ】
【関連項目】 Python, Git バージョン管理システム, Git の利用, 7-Zip
Build Tools for Visual Studio 2022 (ビルドツール for Visual Studio 2022)または Visual Studio 2022 のインストール(Windows 上)
CUDAツールキットは、GPU上でコードをコンパイルするためにC++コンパイラを必要とします。そのため、事前にMicrosoft C++ Build Tools または Visual Studio (C++開発ワークロードを含む) をインストールしておく必要があります。
【インストールの判断】 Build Tools for Visual Studio は,C++コンパイラなどを含む開発ツールセットです. Visual Studio は統合開発環境であり,いくつかのエディションがあり,Build Tools for Visual Studioの機能を含むか連携して使用します.インストールは以下の基準で判断してください:
- コマンドラインからのビルドなど、C++コンパイラ機能のみが必要な場合:
- Visual Studioのエディタやデバッガなどの統合開発環境機能が必要な場合、あるいは、どちらをインストールすべきかよく分からない場合:
Visual Studio Community (または他のエディション) をインストール します.
Visual Studio 2022 をインストールする際に,「C++ によるデスクトップ開発」ワークロードを選択することで,必要なBuild Toolsの機能も一緒にインストールされます.
不明な点がある場合は,Visual Studio 全体をインストール する方が、後で機能を追加する手間が省ける場合があります.
Build Tools for Visual Studio 2022 のインストール(Windows 上)
- Windows で,コマンドプロンプトを管理者権限で起動します(例:Windowsキーを押し,「cmd」と入力し,「管理者として実行」を選択)。
以下の
winget
コマンドを実行します。winget
はWindows標準のパッケージマネージャーです。--scope machine
オプションはシステム全体にインストールすることを意味します。次のコマンドは,Build Tools for Visual Studio 2022と、多くのプログラムで必要とされるVC++ 2015以降の再頒布可能パッケージをインストールします.
- Build Tools for Visual Studio 2022 で C++ によるデスクトップ開発関連コンポーネントのインストール
CUDA開発には、標準のC++開発ツールに加えて、特定のコンポーネントが必要になる場合があります。
- Visual Studio Installer を起動します。
起動方法: スタートメニューから「Visual Studio Installer」を探して実行します.
- Visual Studio Build Tools 2022 の項目で「変更」ボタンをクリックします.
- 「ワークロード」タブで「C++ によるデスクトップ開発」をクリックして選択します。画面右側の「インストールの詳細」で、必要に応じて「v143 ビルドツール用 C++/CLI サポート(最新)」、「ATL」、「MFC」などをチェックします(これらは一般的なC++開発や特定のプロジェクトタイプで必要になる場合があります)。その後、「変更」をクリックしてインストールまたは変更を適用します.
- Visual Studio Installer を起動します。
Visual Studio Community 2022 のインストール(Windows 上)
- Windows で,コマンドプロンプトを管理者権限で起動します。
- インストールコマンドの実行
以下の
winget
コマンドを実行します。--override "--add ..."
部分で、インストールするワークロードやコンポーネントを指定しています。winget install Microsoft.VisualStudio.2022.Community --scope machine --override "--add Microsoft.VisualStudio.Workload.NativeDesktop Microsoft.VisualStudio.ComponentGroup.NativeDesktop.Core Microsoft.VisualStudio.Component.VC.CLI.Support Microsoft.VisualStudio.Component.CoreEditor Microsoft.VisualStudio.Component.NuGet Microsoft.VisualStudio.Component.Roslyn.Compiler Microsoft.VisualStudio.Component.TextTemplating Microsoft.VisualStudio.Component.Windows.SDK.Latest Microsoft.VisualStudio.Component.VC.Tools.x86.x64 Microsoft.VisualStudio.Component.VC.ATL Microsoft.VisualStudio.Component.VC.ATLMFC" winget install Microsoft.VisualStudio.2022.Community --scope machine Microsoft.VCRedist.2015+.x64
インストールされる主要なコンポーネントの説明:
NativeDesktop
(C++によるデスクトップ開発): CUDA開発に必要なC++コンパイラ(VC.Tools.x86.x64
)やWindows SDK (Windows.SDK.Latest
)など、基本的な開発ツール一式を含みます。CoreEditor
: Visual Studioの基本的なコードエディタ機能を提供します。VC.CLI.Support
: C++/CLIを用いた開発サポート(通常、純粋なCUDA C++開発では不要な場合もあります)。NuGet
: .NETライブラリ管理用(C++プロジェクトでも利用されることがあります)。VC.ATL
/VC.ATLMFC
: 特定のWindowsアプリケーション開発フレームワーク(通常、CUDA開発自体には直接必要ありません)。
システム要件と注意事項:
- 管理者権限でのインストールが必須です。
- 必要ディスク容量:10GB以上(選択するコンポーネントにより変動)。
- 推奨メモリ:8GB以上のRAM。
- インストール過程でシステムの再起動が要求される可能性があります。
- 安定したインターネット接続環境が必要です。
後から追加のコンポーネントが必要になった場合は,Visual Studio Installerを使用して個別にインストールすることが可能です.
- インストール完了の確認
インストールが成功したか確認するには、管理者権限のコマンドプロンプトで以下のコマンドを実行します。
winget list Microsoft.VisualStudio.2022.Community
リストに表示されればインストールされています。
トラブルシューティング:
インストール失敗時は,以下のログファイルを確認すると原因究明の手がかりになります:
%TEMP%\dd_setup_
.log %TEMP%\dd_bootstrapper_ .log (
は実行日時に対応する文字列) - (オプション) Visual Studio Installer での確認と変更
winget
でのインストール後も、Visual Studio Installerを使ってインストール内容を確認・変更できます。- Visual Studio Installer を起動します。
- Visual Studio Community 2022 の項目で「変更」をクリックします。
- 「ワークロード」タブで「C++ によるデスクトップ開発」がチェックされていることを確認します。必要であれば、「個別のコンポーネント」タブで特定のツール(例: 特定バージョンのMSVCコンパイラ、CMakeツールなど)を追加・削除できます。「インストールの詳細」で「v143 ビルドツール用 C++/CLI サポート(最新)」などが選択されているかも確認できます。変更後、「変更」または「インストール」をクリックします。
NVIDIA ドライバのインストール(Windows 上)
NVIDIA ドライバとは
NVIDIA ドライバは,NVIDIA製GPUをWindowsシステム上で適切に動作させるための基盤となるソフトウェアです.このドライバをインストールすることにより,GPUの性能を最大限に引き出し,グラフィックス処理はもちろん,CUDAを利用したAI関連アプリケーションなどの計算速度を向上させることが期待できます.
ドライバは通常、NVIDIA公式サイトからダウンロードするか、NVIDIA GeForce Experienceソフトウェアを通じてインストール・更新します。
公式サイト: https://www.nvidia.co.jp/Download/index.aspx?lang=jp
【サイト内の関連ページ】
- (再掲) NVIDIA グラフィックス・ボードの確認
インストールするドライバを選択するために、まずご使用のPCに搭載されているNVIDIAグラフィックス・ボードの種類を確認します。(確認済みであれば、この手順は不要です。) Windows のコマンドプロンプトで次のコマンドを実行します。
wmic path win32_VideoController get name
- NVIDIA ドライバのダウンロード
確認したグラフィックス・ボードのモデル名と、お使いのWindowsのバージョン(例: Windows 11, Windows 10 64-bit)に対応するドライバを、以下のNVIDIA公式サイトからダウンロードします.
https://www.nvidia.co.jp/Download/index.aspx?lang=jp
サイトの指示に従い、製品タイプ、製品シリーズ、製品ファミリー、OS、言語などを選択して検索し、適切なドライバ(通常は最新のGame Ready ドライバまたはStudio ドライバ)をダウンロードします。
- ドライバのインストール
ダウンロードしたインストーラー(.exeファイル)を実行し、画面の指示に従ってインストールを進めます。「カスタムインストール」を選択すると、インストールするコンポーネント(ドライバ本体、GeForce Experience、PhysXなど)を選ぶことができます。通常は「高速(推奨)」で問題ありません。
インストール完了後、システムの再起動を求められる場合があります。
- CUDA対応のNVIDIA GPU。
- 対応するNVIDIA ドライバ。
- サポートされているバージョンのC++コンパイラ (Visual StudioまたはBuild Toolsをインストール済み)。
- Windows では,NVIDIA CUDA ツールキットのインストール中は,予期せぬ問題を避けるため、なるべく他のアプリケーションは終了しておくことが推奨されます。
- インストール後に環境変数が正しく設定されているか確認することが重要です。
- NVIDIA CUDA ツールキットのアーカイブの公式ページ: https://developer.nvidia.com/cuda-toolkit-archive (他のバージョンが必要な場合)
- NVIDIA CUDA ツールキット の公式ドキュメント: https://docs.nvidia.com/cuda/index.html
- NVIDIA CUDA ツールキットのインストールに関する,NVIDIA CUDA Installation Guide for Windows: https://docs.nvidia.com/cuda/cuda-installation-guide-windows/index.html
- (再掲) 他のウィンドウを閉じる:インストール中のコンフリクトを避けるため、可能な限り他のアプリケーションを終了します。
- Windows で,コマンドプロンプトを管理者権限で起動します。
-
winget
コマンドで CUDA 11.8 をインストールします。以下のコマンドは、(必要であれば)NVIDIA GeForce Experienceと、指定したバージョンのNVIDIA CUDA ツールキット (11.8) をインストールします。また、
CUDA_HOME
環境変数を設定します(一部のツールで参照されることがあります)。rem グラフィックボードの確認 (参考) wmic path win32_VideoController get name rem CUDA Toolkit 11.8 のインストール winget install --scope machine Nvidia.CUDA --version 11.8 rem CUDA_HOME 環境変数の設定 (システム環境変数として設定) powershell -command "[System.Environment]::SetEnvironmentVariable(\"CUDA_HOME\", \"C:\Program Files\NVIDIA GPU Computing Toolkit\CUDA\v11.8\", \"Machine\")"
注釈: これは特定のバージョン(11.8)をインストールする例です。他のバージョンをインストールする場合は
--version
オプションを適宜変更してください(例:--version 11.2
)。利用可能なバージョンはwinget search Nvidia.CUDA
で確認できます。 - (重要) ユーザ環境変数 TEMP の設定(日本語ユーザ名の場合)
Windows のユーザ名に日本語(マルチバイト文字)が含まれている場合、CUDAコンパイラ
nvcc
が一時ファイルの作成に失敗し、コンパイルが正常に動作しないことがあります(エラーメッセージが表示されない場合もあるため注意が必要です)。この問題を回避するために、ユーザ環境変数TEMP
およびTMP
を、ASCII文字のみのパス(例:C:\TEMP
)に変更します。管理者権限のコマンドプロンプトで,次のコマンドを実行して
C:\TEMP
ディレクトリを作成し、ユーザ環境変数TEMP
とTMP
を設定します。mkdir C:\TEMP powershell -command "[System.Environment]::SetEnvironmentVariable(\"TEMP\", \"C:\TEMP\", \"User\")" powershell -command "[System.Environment]::SetEnvironmentVariable(\"TMP\", \"C:\TEMP\", \"User\")"
この設定は、コマンドプロンプトを再起動するか、Windowsに再サインインした後に有効になります。
- NVIDIA cuDNN の公式ページ(ダウンロードにはDeveloper Programへの登録が必要): https://developer.nvidia.com/cudnn
- NVIDIA Developer Program メンバーシップへの加入: cuDNNのダウンロードには無料のメンバーシップ登録が必要です。
NVIDIA Developer Program の公式ページ: https://developer.nvidia.com/developer-program
- 互換バージョンの選択とダウンロード: インストール済みのCUDAツールキットのバージョン (今回は11.x) に適合するcuDNNのバージョン (今回はv8.9.7) を選択し、Windows用のzipファイルをダウンロードします。
- ファイルの展開と配置: ダウンロードしたzipファイルを展開(解凍)し、中のファイル(
bin
,include
,lib
フォルダ内)を、CUDAツールキットのインストールディレクトリにコピーします。 - (オプション) 環境変数の設定: 必要に応じてシステム環境変数
CUDNN_PATH
を設定します。 - (必要に応じて) ZLIB DLL のインストール:
zlibwapi.dll
が見つからないエラーが発生する場合にインストールします。 - 動作確認: cuDNNライブラリ (
cudnn64_*.dll
) にパスが通っているか確認します。 - Windows で,管理者権限でコマンドプロンプトを起動(手順:Windowsキーまたはスタートメニュー >
cmd
と入力 > 右クリック > 「管理者として実行」)。 - 次のコマンドを実行
次のコマンドは,zlibをインストールし,パスを通すものである.
cd /d c:%HOMEPATH% rmdir /s /q zlib git clone https://github.com/madler/zlib cd zlib del CMakeCache.txt rmdir /s /q CMakeFiles\ cmake . -G "Visual Studio 17 2022" -A x64 -T host=x64 -DCMAKE_INSTALL_PREFIX=c:/zlib cmake --build . --config RELEASE --target INSTALL powershell -command "$oldpath = [System.Environment]::GetEnvironmentVariable(\"Path\", \"Machine\"); $oldpath += \";c:\zlib\bin\"; [System.Environment]::SetEnvironmentVariable(\"Path\", $oldpath, \"Machine\")" powershell -command "[System.Environment]::SetEnvironmentVariable(\"ZLIB_HOME\", \"C:\zlib\", \"Machine\")"
- zlib の公式ページ: https://www.zlib.net/
- NVIDIA cuDNN のウェブページを開く
- ダウンロードしたいので,cuDNNのところにある「Download cuDNN」をクリック.
- cuDNN Downloads のページで「Archive of Previous Releases」をクリック
- 「cuDNN 8.x - 1.x」をクリック
- ダウンロードしたいバージョンを選ぶ
ここでは「NVIDIA cuDNN v8.9.7 for CUDA 11.x」を選んでいる.
このとき,画面の「for CUDA ...」のところを確認し,使用するNVIDIA CUDA のバージョンに合うものを選ぶこと.
- Windows にインストールするので Windows 版を選ぶ
- NVIDIA Developer Program メンバーシップに入る
NVIDIA cuDNN のダウンロードのため.
「Join now」をクリック.その後,画面の指示に従う. 利用者本人が,電子メールアドレス,表示名,パスワード,生年月日を登録.利用条件等に合意.
- ログインする
- 調査の画面が出たときは,調査に応じる
- ライセンス条項の確認
- ダウンロードが始まる.
- ダウンロードした .zip ファイルを展開(解凍)する.
その中のサブディレクトリを確認しておく.
- NVIDIA CUDA ツールキットをインストールしたディレクトリを確認する.「C:\Program Files\NVIDIA GPU Computing Toolkit\CUDA\v11.8」のようになっている.
- 確認したら,
さきほど展開してできたすべてのファイルとディレクトリを,NVIDIA CUDA ツールキットをインストールしたディレクトリにコピーする
- パスが通っていることを確認.
次の操作により,cudnn64_8.dll にパスが通っていることを確認する.
Windows のコマンドプロンプトを開き,次のコマンドを実行する.エラーメッセージが出ないことを確認.
where cudnn64_8.dll
- Windows の システム環境変数 CUDNN_PATH の設定を行う.
Windows では,
コマンドプロンプトを管理者として開き,
次のコマンドを実行することにより,
システム環境変数 CUDNN_PATH の設定を行うことができる.
コマンドプロンプトを管理者として実行: 別ページ »で説明
powershell -command "[System.Environment]::SetEnvironmentVariable(\"CUDNN_PATH\", \"C:\Program Files\NVIDIA GPU Computing Toolkit\CUDA\v11.8\", \"Machine\")"
- Windows で,管理者権限でコマンドプロンプトを起動(手順:Windowsキーまたはスタートメニュー >
cmd
と入力 > 右クリック > 「管理者として実行」)。 - TensorFlow 2.10.1 のインストール(Windows 上)
次のコマンドを実行することにより,TensorFlow 2.10.1 および関連パッケージ(tf_slim,tensorflow_datasets,tensorflow-hub,Keras,keras-tuner,keras-visualizer)がインストール(インストール済みのときは最新版に更新)される. そして,Pythonパッケージ(Pillow, pydot, matplotlib, seaborn, pandas, scipy, scikit-learn, scikit-learn-intelex, opencv-python, opencv-contrib-python)がインストール(インストール済みのときは最新版に更新)される.
python -m pip uninstall -y protobuf tensorflow tensorflow-cpu tensorflow-gpu tensorflow-intel tensorflow-text tensorflow-estimator tf-models-official tf_slim tensorflow_datasets tensorflow-hub keras keras-tuner keras-visualizer python -m pip install -U protobuf tensorflow==2.10.1 tf_slim tensorflow_datasets==4.8.3 tensorflow-hub tf-keras keras keras_cv keras-tuner keras-visualizer python -m pip install git+https://github.com/tensorflow/docs python -m pip install git+https://github.com/tensorflow/examples.git python -m pip install git+https://www.github.com/keras-team/keras-contrib.git python -m pip install -U pillow pydot matplotlib seaborn pandas scipy scikit-learn scikit-learn-intelex opencv-python opencv-contrib-python
- コマンドプロンプトを管理者として開く.
コマンドプロンプトを管理者として実行: 別ページ »で説明
- protoc のダウンロードと展開(解凍)
「3.19.4」のところにはバージョンを指定すること.
python -m pip install -U protobuf==3.19.6c:\protoc cd c:\protoc curl -L -O https://github.com/protocolbuffers/protobuf/releases/download/v23.2/protoc-23.2-win64.zip powershell -command "Expand-Archive -DestinationPath . -Path protoc-23.2-win64.zip"
- Windows の システム環境変数 Pathに,c:\protoc\bin を追加することにより,パスを通す.
Windows で,管理者権限でコマンドプロンプトを起動(手順:Windowsキーまたはスタートメニュー >
cmd
と入力 > 右クリック > 「管理者として実行」)。powershell -command "$oldpath = [System.Environment]::GetEnvironmentVariable(\"Path\", \"Machine\"); $oldpath += \";c:\protoc\bin\"; [System.Environment]::SetEnvironmentVariable(\"Path\", $oldpath, \"Machine\")"
- Windows で,管理者権限でコマンドプロンプトを起動(手順:Windowsキーまたはスタートメニュー >
cmd
と入力 > 右クリック > 「管理者として実行」)。 - pycocotools, COCO 2018 Panoptic Segmentation Task API のインストール
COCOデータセットをPythonで扱うためには,pycocotools を使う. pycocotools を使うことで,COCOデータセットのアノテーションを扱いやすくなる.
次のコマンドを実行
python -m pip install -U --ignore-installed pip python -m pip install -U cython wheel python -m pip install "git+https://github.com/philferriere/cocoapi.git#egg=pycocotools&subdirectory=PythonAPI" python -m pip install git+https://github.com/cocodataset/panopticapi.git
- コマンドプロンプトを管理者として開く.
- Deeplab2 のダウンロード
rmdir /s /q c:\deeplab2 mkdir c:\deeplab2 cd c:\deeplab2 git clone https://github.com/google-research/deeplab2.git
- protoc を用いてコンパイル
cd c:\deeplab2 protoc deeplab2\*.proto --python_out=.
- Windows の システム環境変数 PYTHONPATHに,c:\deeplab2 を追加することにより,パスを通す.
Windows で,管理者権限でコマンドプロンプトを起動(手順:Windowsキーまたはスタートメニュー >
cmd
と入力 > 右クリック > 「管理者として実行」)。powershell -command "$oldpath = [System.Environment]::GetEnvironmentVariable(\"PYTHONPATH\", \"Machine\"); $oldpath += \";c:\deeplab2\"; [System.Environment]::SetEnvironmentVariable(\"PYTHONPATH\", $oldpath, \"Machine\")"
- Windows で,管理者権限でコマンドプロンプトを起動(手順:Windowsキーまたはスタートメニュー >
cmd
と入力 > 右クリック > 「管理者として実行」)。 - TensorFlow モデルのダウンロード,プロトコル・バッファ・コンパイラ (protocol buffer compiler) によるコンパイル
cd /d c:%HOMEPATH% rmdir /s /q models git clone https://github.com/tensorflow/models.git cd models python -m pip install -r official/requirements.txt python -m pip install -U opencv-python cd /d c:%HOMEPATH%\models\research protoc object_detection\protos\*.proto --python_out=. protoc lstm_object_detection\protos\*.proto --python_out=. cd /d c:%HOMEPATH%\models\research\delf protoc delf\protos\*.proto --python_out=.
- TensorFlow モデルの unified_detector のインストール
cd /d c:%HOMEPATH%\models python -m pip install -r official\projects\unified_detector\requirements.txt
- 学習済みモデルのダウンロード
- 次のページで「Download the checkpoint」のところで「ckpt」をクリック
https://github.com/tensorflow/models/tree/master/official/projects/unified_detector
- ダウンロードしたファイルは,%HOMEPATH%\models に置く
- 次のコマンドを実行することにより,展開(解凍)する.
cd /d c:%HOMEPATH%\models move vision_unified_detector_unified_detector_ckpt.gz vision_unified_detector_unified_detector_ckpt.tar.gz "c:\Program Files\7-Zip\7z.exe" x vision_unified_detector_unified_detector_ckpt.tar.gz "c:\Program Files\7-Zip\7z.exe" x vision_unified_detector_unified_detector_ckpt.tar dir
- ckpt.data-00000-of-00001, ckpt.index ができるので確認
- 次のページで「Download the checkpoint」のところで「ckpt」をクリック
https://github.com/tensorflow/models/tree/master/official/projects/unified_detector
- 画像ファイルを準備
画像ファイル名は demo.png であるとする
- 動作確認のため,テキスト検出を行ってみる
cd /d c:%HOMEPATH% cd models mkdir vis python -m official.projects.unified_detector.run_inference ^ --gin_file=official/projects/unified_detector/configs/gin_files/unified_detector_model.gin ^ --ckpt_path=.\ckpt ^ --img_file=demo.png ^ --output_path=./demo.json ^ --vis_dir=vis
- 結果を表示
cd vis demo-line.jpg demo-paragraph.jpg demo-word.jpg
単語単位のテキスト検出
行単位のテキスト検出
段落単位のテキスト検出
- 別の画像で試した場合
単語単位のテキスト検出
行単位のテキスト検出
段落単位のテキスト検出
- 公式のプログラムを書き換えた次のプログラムを使用.run.py のようなファイル名で,
%HOMEPATH%\models に保存
# Copyright 2023 The TensorFlow Authors. All Rights Reserved. # # Licensed under the Apache License, Version 2.0 (the "License"); # you may not use this file except in compliance with the License. # You may obtain a copy of the License at # # http://www.apache.org/licenses/LICENSE-2.0 # # Unless required by applicable law or agreed to in writing, software # distributed under the License is distributed on an "AS IS" BASIS, # WITHOUT WARRANTIES OR CONDITIONS OF ANY KIND, either express or implied. # See the License for the specific language governing permissions and # limitations under the License. r"""A binary to run unified detector.""" import json import os from typing import Any, Dict, Sequence, Union from absl import app from absl import flags from absl import logging import cv2 import gin import numpy as np import tensorflow as tf import tqdm from official.projects.unified_detector import external_configurables # pylint: disable=unused-import from official.projects.unified_detector.modeling import universal_detector from official.projects.unified_detector.utils import utilities # group two lines into a paragraph if affinity score higher than this _PARA_GROUP_THR = 0.5 # MODEL spec _GIN_FILE = flags.DEFINE_string( 'gin_file', None, 'Path to the Gin file that defines the model.') _CKPT_PATH = flags.DEFINE_string( 'ckpt_path', None, 'Path to the checkpoint directory.') _IMG_SIZE = flags.DEFINE_integer( 'img_size', 1024, 'Size of the image fed to the model.') # Output _OUTPUT_PATH = flags.DEFINE_string('output_path', None, 'Path for the output.') def _preprocess(raw_image: np.ndarray) -> Union[np.ndarray, float]: """Convert a raw image to properly resized, padded, and normalized ndarray.""" # (1) convert to tf.Tensor and float32. img_tensor = tf.convert_to_tensor(raw_image, dtype=tf.float32) # (2) pad to square. height, width = img_tensor.shape[:2] maximum_side = tf.maximum(height, width) height_pad = maximum_side - height width_pad = maximum_side - width img_tensor = tf.pad( img_tensor, [[0, height_pad], [0, width_pad], [0, 0]], constant_values=127) ratio = maximum_side / _IMG_SIZE.value # (3) resize long side to the maximum length. img_tensor = tf.image.resize( img_tensor, (_IMG_SIZE.value, _IMG_SIZE.value)) img_tensor = tf.cast(img_tensor, tf.uint8) # (4) normalize img_tensor = utilities.normalize_image_to_range(img_tensor) # (5) Add batch dimension and return as numpy array. return tf.expand_dims(img_tensor, 0).numpy(), float(ratio) def load_model() -> tf.keras.layers.Layer: gin.parse_config_file(_GIN_FILE.value) model = universal_detector.UniversalDetector() ckpt = tf.train.Checkpoint(model=model) ckpt_path = _CKPT_PATH.value logging.info('Load ckpt from: %s', ckpt_path) ckpt.restore(ckpt_path).expect_partial() return model def inference(img, model: tf.keras.layers.Layer) -> Dict[str, Any]: """Inference step.""" img_ndarray, ratio = _preprocess(img) output_dict = model.serve(img_ndarray) class_tensor = output_dict['classes'].numpy() mask_tensor = output_dict['masks'].numpy() group_tensor = output_dict['groups'].numpy() indices = np.where(class_tensor[0])[0].tolist() # indices of positive slots. mask_list = [ mask_tensor[0, :, :, index] for index in indices] # List of mask ndarray. # Form lines and words lines = [] line_indices = [] for index, mask in tqdm.tqdm(zip(indices, mask_list)): line = { 'words': [], 'text': '', } contours, _ = cv2.findContours( (mask > 0.).astype(np.uint8), cv2.RETR_TREE, cv2.CHAIN_APPROX_SIMPLE)[-2:] for contour in contours: if (isinstance(contour, np.ndarray) and len(contour.shape) == 3 and contour.shape[0] > 2 and contour.shape[1] == 1 and contour.shape[2] == 2): cnt_list = (contour[:, 0] * ratio).astype(np.int32).tolist() line['words'].append({'text': '', 'vertices': cnt_list}) else: logging.error('Invalid contour: %s, discarded', str(contour)) if line['words']: lines.append(line) line_indices.append(index) # Form paragraphs line_grouping = utilities.DisjointSet(len(line_indices)) affinity = group_tensor[0][line_indices][:, line_indices] for i1, i2 in zip(*np.where(affinity > _PARA_GROUP_THR)): line_grouping.union(i1, i2) line_groups = line_grouping.to_group() paragraphs = [] for line_group in line_groups: paragraph = {'lines': []} for id_ in line_group: paragraph['lines'].append(lines[id_]) if paragraph: paragraphs.append(paragraph) return paragraphs def main(argv: Sequence[str]) -> None: if len(argv) > 1: raise app.UsageError('Too many command-line arguments.') model = load_model() output = {'annotations': []} v = cv2.VideoCapture(0) while(v.isOpened()): r, f = v.read() if ( r == False ): break cv2.imshow("", f) img = cv2.cvtColor(f, cv2.COLOR_BGR2RGB) output['annotations'].append({ 'image_id': 'videocam', 'paragraphs': inference(img, model), }) key = output['annotations'][-1]['image_id'] paragraphs = output['annotations'][-1]['paragraphs'] word_bnds = [] line_bnds = [] para_bnds = [] for paragraph in paragraphs: paragraph_points_list = [] for line in paragraph['lines']: line_points_list = [] for word in line['words']: word_bnds.append( np.array(word['vertices'], np.int32).reshape((-1, 1, 2))) line_points_list.extend(word['vertices']) paragraph_points_list.extend(line_points_list) line_points = np.array(line_points_list, np.int32) # (N,2) left = int(np.min(line_points[:, 0])) top = int(np.min(line_points[:, 1])) right = int(np.max(line_points[:, 0])) bottom = int(np.max(line_points[:, 1])) line_bnds.append( np.array([[[left, top]], [[right, top]], [[right, bottom]], [[left, bottom]]], np.int32)) para_points = np.array(paragraph_points_list, np.int32) # (N,2) left = int(np.min(para_points[:, 0])) top = int(np.min(para_points[:, 1])) right = int(np.max(para_points[:, 0])) bottom = int(np.max(para_points[:, 1])) para_bnds.append( np.array([[[left, top]], [[right, top]], [[right, bottom]], [[left, bottom]]], np.int32)) for name, bnds in zip(['paragraph', 'line', 'word'], [para_bnds, line_bnds, word_bnds]): vis = cv2.polylines(img, bnds, True, (0, 0, 255), 2) cv2.imshow("", cv2.cvtColor(vis, cv2.COLOR_RGB2BGR)) # Press Q to exit if cv2.waitKey(1) & 0xFF == ord('q'): break v.release() cv2.destroyAllWindows() with tf.io.gfile.GFile(_OUTPUT_PATH.value, mode='w') as f: f.write(json.dumps(output, ensure_ascii=False, indent=2)) if __name__ == '__main__': flags.mark_flags_as_required(['gin_file', 'ckpt_path', 'output_path']) app.run(main)
- 次のコマンドを実行
cd /d c:%HOMEPATH% cd models python run.py ^ --gin_file=official/projects/unified_detector/configs/gin_files/unified_detector_model.gin ^ --ckpt_path=.\ckpt ^ --output_path=./demo.json
NVIDIA CUDA ツールキット 11.8 のインストール(Windows 上)
CUDAツールキットには、GPUでプログラムを実行するためのライブラリ、`nvcc`コンパイラ、開発ツールなどが含まれています。ここでは`winget`を使ってCUDA 11.8をインストールする手順を示します。
NVIDIA CUDA ツールキットの概要と注意点
NVIDIAのGPUを使用して並列計算を行うための開発・実行環境です。
主な機能: GPU を利用した並列処理のコンパイルと実行、GPU のメモリ管理、C++をベースとした拡張言語(CUDA C/C++)とAPI、ライブラリ(cuBLAS, cuFFTなど)を提供します。
【NVIDIA CUDA ツールキットの動作に必要なもの】
【Windows でインストールするときの一般的な注意点】
【関連する外部ページ】
【関連項目】 NVIDIA CUDA ツールキットの概要, NVIDIA CUDA ツールキットの他バージョンのインストール
NVIDIA cuDNN 8.9.7 のインストール(Windows 上)
NVIDIA cuDNN
NVIDIA cuDNN は,NVIDIA CUDA ツールキット上で動作する、高性能なディープラーニング用ライブラリです.畳み込みニューラルネットワーク (CNN) やリカレントニューラルネットワーク (RNN) など,さまざまなディープラーニングモデルのトレーニングと推論を高速化します.
【cuDNN利用時の注意点: zlibwapi.dll エラー】
Windows環境でcuDNNを利用するアプリケーションを実行した際に、「Could not locate zlibwapi.dll. Please make sure it is in your library path!」というエラーが表示されることがあります。これは、cuDNNの一部の機能が圧縮ライブラリである zlib
に依存しているためです。このエラーが発生した場合は、後述する手順で ZLIB DLL をインストールする必要があります。
【関連する外部ページ】
NVIDIA cuDNN のインストール(Windows 上)の概要
zlib のインストール(Windows 上)
【関連する外部ページ】
【関連項目】 zlib
NVIDIA cuDNN 8.9.7 のインストール(Windows 上)
TensorFlow 2.10.1 のインストール(Windows 上)
Unified Scene Text Detection のインストールとテスト実行(Windows 上)
プロトコル・バッファ・コンパイラ (protocol buffer compiler) のインストール(Windows 上)
プロトコル・バッファ・コンパイラ (protocol buffer compiler) のインストールを行う.
pycocotools のインストール(Windows 上)
pycocotools のインストールを行う.
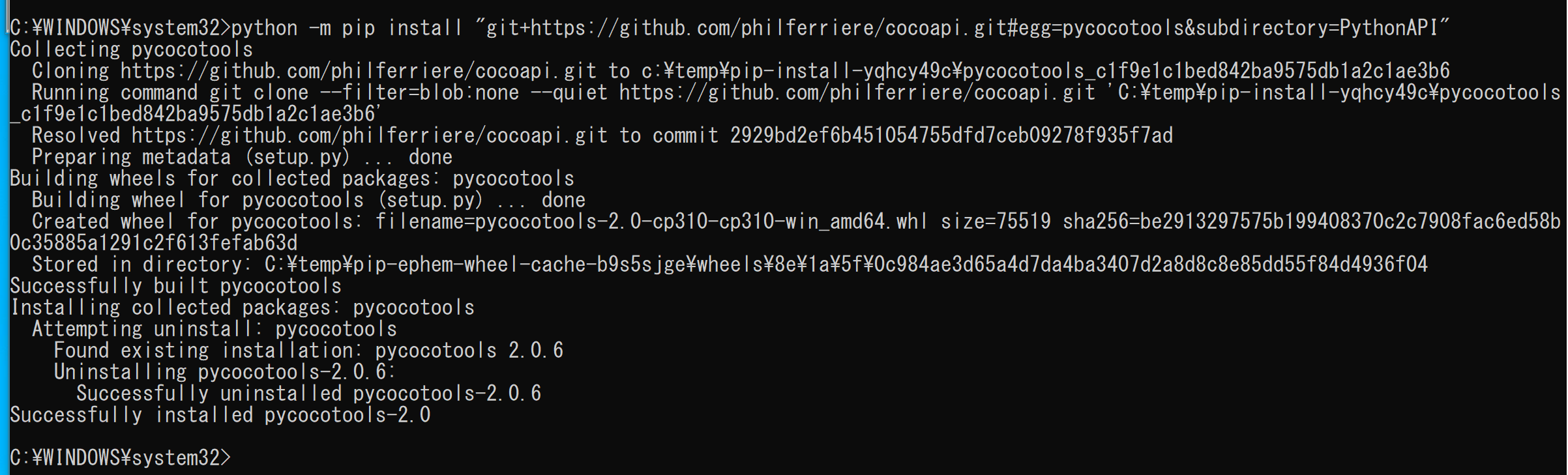
Deeplab2 のインストール(Windows 上)
DeepLab2 のインストールを行う.