階層クラスタリング(数値ベクトルの集合)(a set of numeric vectors)
サンプルデータ
* サンプルデータ2次元
- cluster #1: center(0,0), N=100, vec=(1,0)
- cluster #2: center(3,4), N=200, vec=(2,-1)
require(data.table)
require(ggplot2)
x1 <- rnorm(100)
y1 <- rnorm(100) * 0.2
a2 <- rnorm(200)
b2 <- rnorm(200) * 0.4
x2 <- a2 * 2 + b2 + 3
y2 <- - a2 + 2 * b2 + 4
D <- data.table( x=c(x1,x2), y=c(y1,y2) )
p <- ggplot(D, aes(x = x, y = y))
p + geom_point(size = 2)
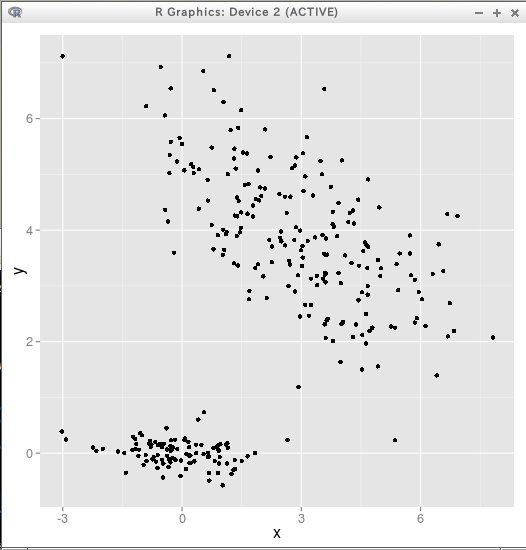
* サンプルデータ6次元
require(data.table)
require(ggplot2)
require(mvtnorm)
T <- data.table( rbind(rmvnorm(200, rep(0, 6), diag(c(5, rep(1,5)))),
rmvnorm( 15, c(0, rep(20, 5)), diag(rep(1, 6)))) )
plot(T)
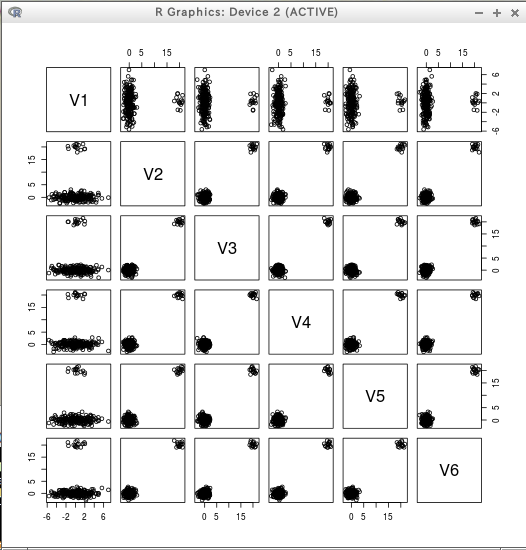
階層クラスタリング (hierarchical clustering)
mclust パッケージの Mclust() 関数を用いたクラスタリング (clusering using Mclust() in the mclust package)
- 準備
install.packages("mclust")
- クラスタリング結果のプロット (plot clustering result)
* テストデータ
require(mclust) C = Mclust(D) summary(C) plot(C)
require(mclust) C2 = Mclust(T) summary(C2) plot(C2)
* iris
require(mclust) C3 = Mclust(iris[,c(1:4)]) summary(C3) plot(C3)
- クラスタリング結果を整数ベクトルで得る (get clustering result)
* テストデータ
C$classification C$uncertainty
C2$classification C2$uncertainty
* iris
C3$classification C3$uncertainty
- 元データとクラスタに関する属性の表示
- n : データの観測数 (The number of observations in the data.)
- d : データの次元 (The dimension of the data.)
- G : 最適なクラスタ数 (The optimal number of mixture components.)
* テストデータ
C$d C$n C$G
C2$d C2$n C2$G
* iris
C3$d C3$n C3$G
- Mclust VVV モデル (ellipsoidal, varying volume, shape, and orientation) model) の各クラスタの属性の表示
- pro : クラスタの混合率 (A vector whose kth component is the mixing proportion for the kth component of the mixture model. If missing, equal proportions are assumed.)
- mean : クラスタの平均 (The mean for each component. If there is more than one component, this is a matrix whose kth column is the mean of the kth component of the mixture model.)
- variance$d : d はデータの次元 (The dimension of the data.)
- variance$G : G はクラスタ数 (The number of components in the mixture model.)
- variance$sigma : d かける d かける G の行列. [,,k]はk番目のクラスタの分散共分散行列 (For all multidimensional mixture models. A d by d by G matrix array whose [,,k]th entry is the covariance matrix for the kth component of the mixture model.)
- variance$cholsigma :
d かける d かける G の行列. [,,k]はk番目のクラスタの上三角 Cholesky factor
(For the unconstrained covariance mixture model "VVV". A d by d by G matrix array whose [,,k]th entry is the upper triangular Cholesky factor of the covariance matrix for the kth component of the mixture model.)
* テストデータ
C$parameters$pro C$parameters$mean C$parameters$variance$d C$parameters$variance$G C$parameters$variance$sigma C$parameters$variance$cholsigma
C2$parameters$pro C2$parameters$mean C2$parameters$variance$d C2$parameters$variance$G C2$parameters$variance$sigma C2$parameters$variance$cholsigma
* iris
C3$parameters$pro C3$parameters$mean C3$parameters$variance$d C3$parameters$variance$G C3$parameters$variance$sigma C3$parameters$variance$cholsigma
確認
sd(x1) sd(y1) sd(x2) sd(y2) sqrt( C$parameters$variance$sigma )
階層クラスタリングと clPairs によるプロット
* テストデータ
hcTree <- hc(modelName = "VVV", data = D)
cl <- hclass(hcTree, c(2,3,4,5))
##
par(pty = "s", mfrow = c(1,1))
clPairs(D, cl=cl[,"2"])
clPairs(D, cl=cl[,"5"])
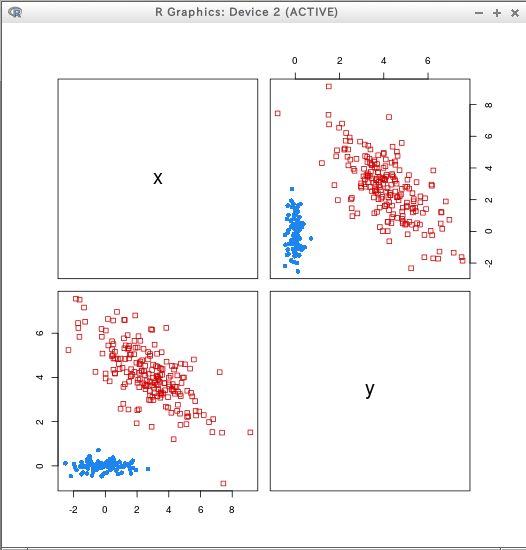
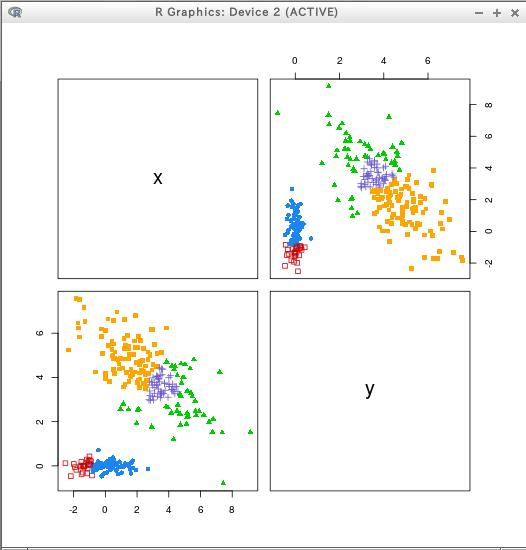
hcTree <- hc(modelName = "VVV", data = T)
cl <- hclass(hcTree, c(2,3,4,5))
##
par(pty = "s", mfrow = c(1,1))
clPairs(T, cl=cl[,"2"])
clPairs(T, cl=cl[,"5"])
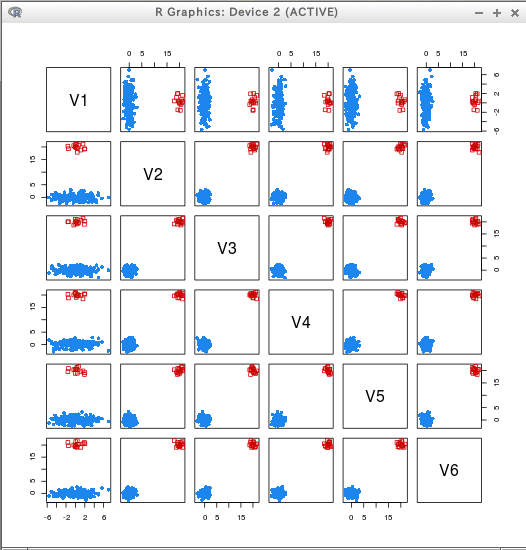
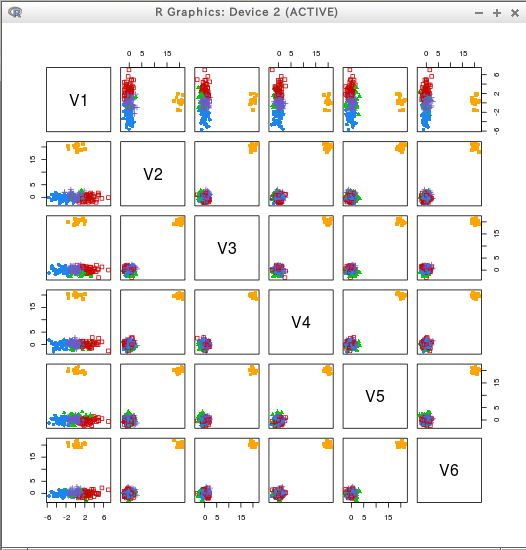
* iris
hcTree <- hc(modelName = "VVV", data = iris[,c(1:4)])
cl <- hclass(hcTree,c(2,3,4,5))
## Not run:
par(pty = "s", mfrow = c(1,1))
clPairs(iris[,c(1:4)],cl=cl[,"2"])
clPairs(iris[,c(1:4)],cl=cl[,"5"])
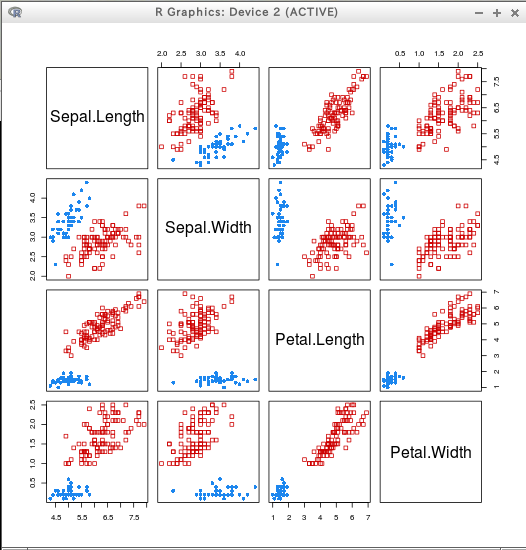
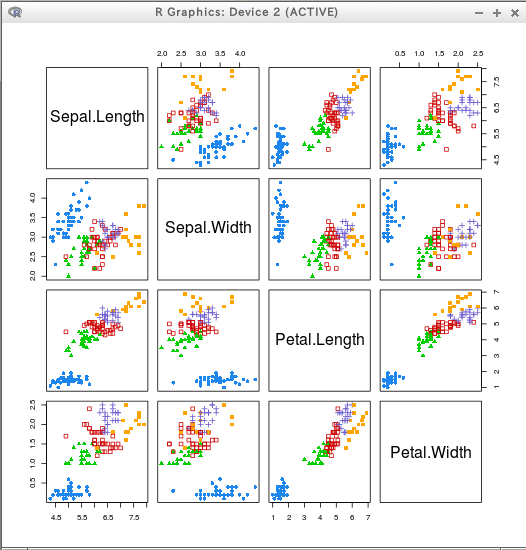
階層クラスタリングと coordProj によるプロット
* テストデータ
hcTree <- hc(modelName = "VVV", data = D)
cl <- hclass(hcTree, c(2,3,4,5))
par(mfrow = c(1,2))
dimens <- c(1,2)
coordProj(D, dimens = dimens, classification=cl[,"2"])
coordProj(D, dimens = dimens, classification=cl[,"5"])
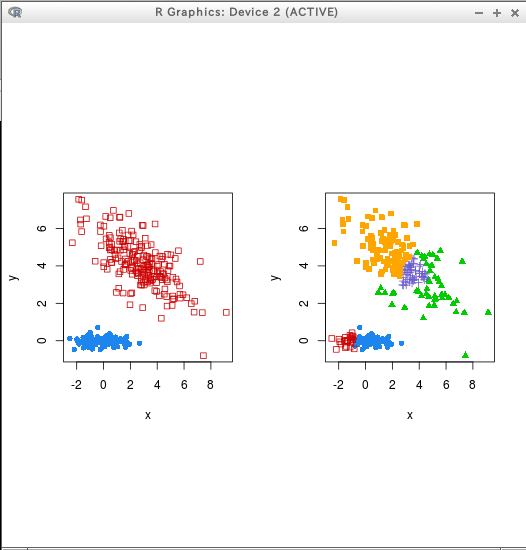
hcTree <- hc(modelName = "VVV", data = T)
cl <- hclass(hcTree, c(2,3,4,5))
par(mfrow = c(1,2))
dimens <- c(1,2)
coordProj(T, dimens = dimens, classification=cl[,"2"])
coordProj(T, dimens = dimens, classification=cl[,"5"])
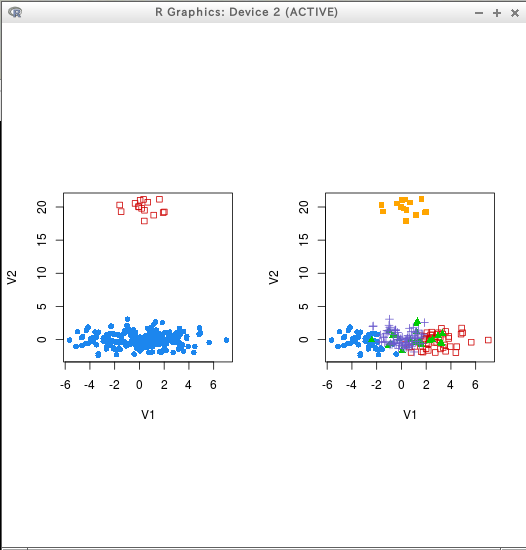
* iris
hcTree <- hc(modelName = "VVV", data = iris[,c(1:4)])
cl <- hclass(hcTree, c(2,3,4,5))
par(mfrow = c(1,2))
dimens <- c(1,2)
coordProj(iris[,c(1:4)], dimens = dimens, classification=cl[,"2"])
coordProj(iris[,c(1:4)], dimens = dimens, classification=cl[,"5"])
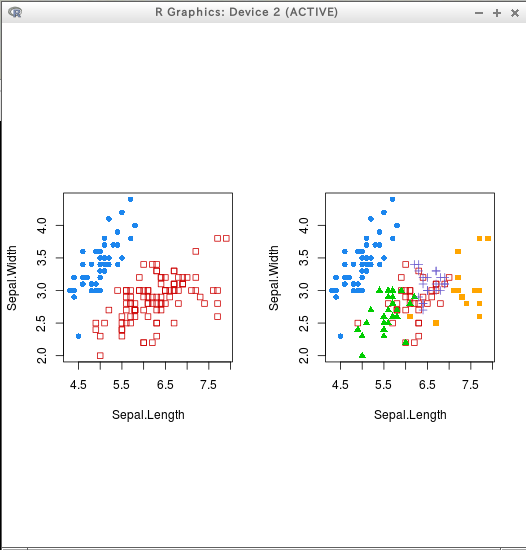
density plot
* テストデータ
require(mclust)
dens = densityMclust(D)
plot(dens)
plot(dens, D)
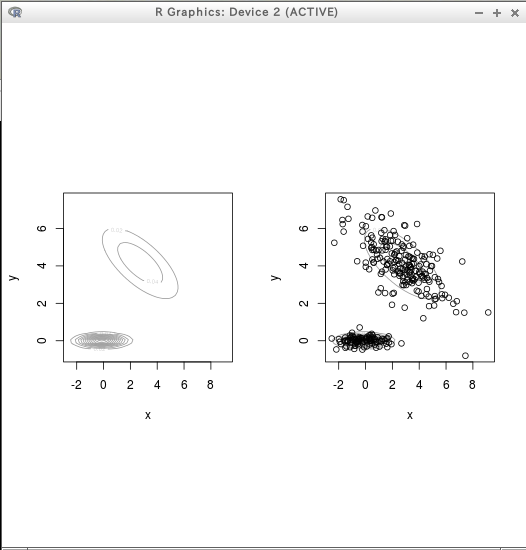
require(mclust)
dens = densityMclust(T)
plot(dens)
plot(dens, T)
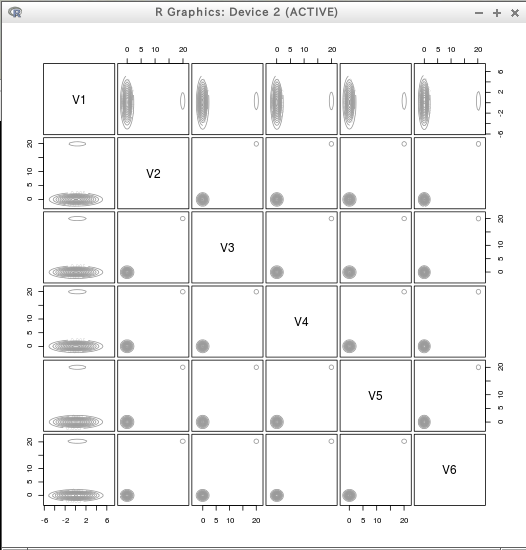
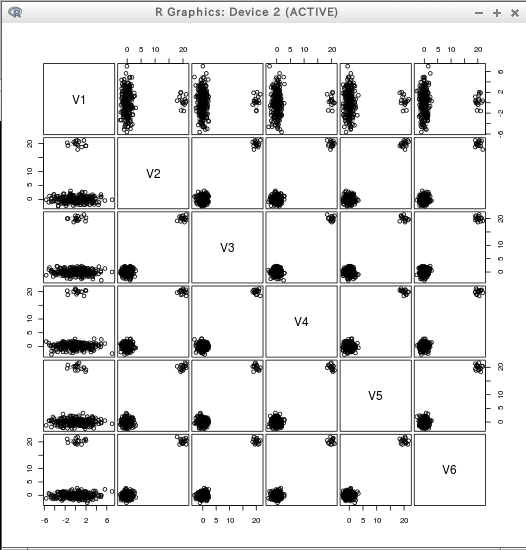
* iris
require(mclust)
dens = densityMclust(iris[,1:4])
plot(dens)
plot(dens, iris[,1:4])
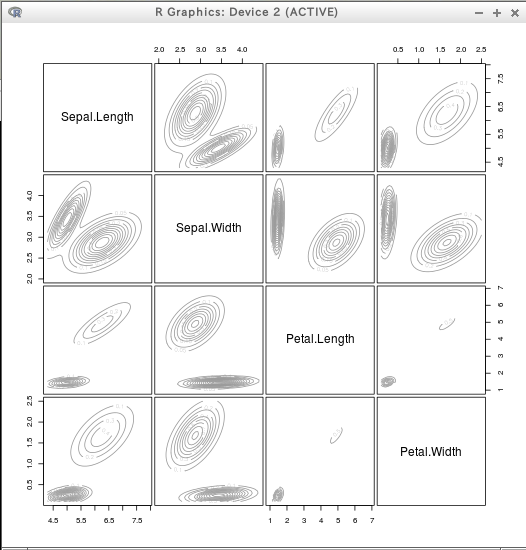
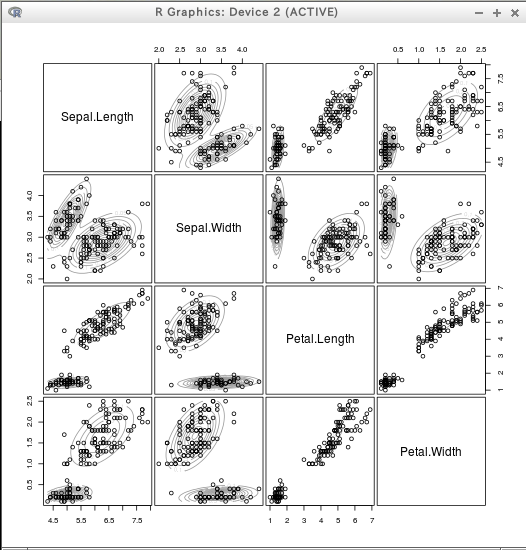
density plot 色付き
* テストデータ
require(mclust)
C = Mclust(D)
dens = densityMclust(D)
plot(dens)
plot(dens, D, col = "grey",
points.col = mclust.options()$classPlotColors[C$classification],
pch = C$classification)
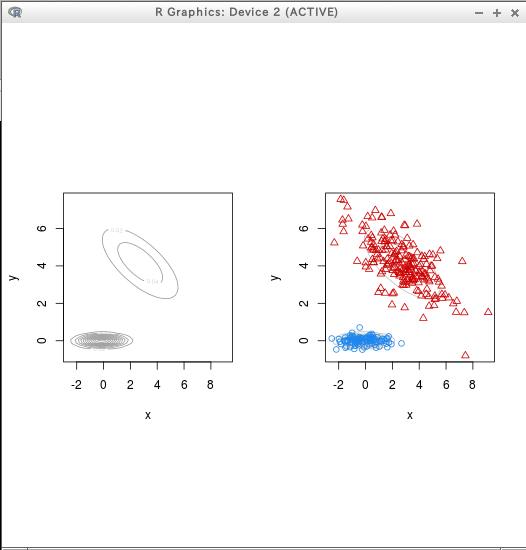
require(mclust)
C2 = Mclust(T)
dens = densityMclust(T)
plot(dens, T, col = "grey",
points.col = mclust.options()$classPlotColors[C2$classification],
pch = C2$classification)
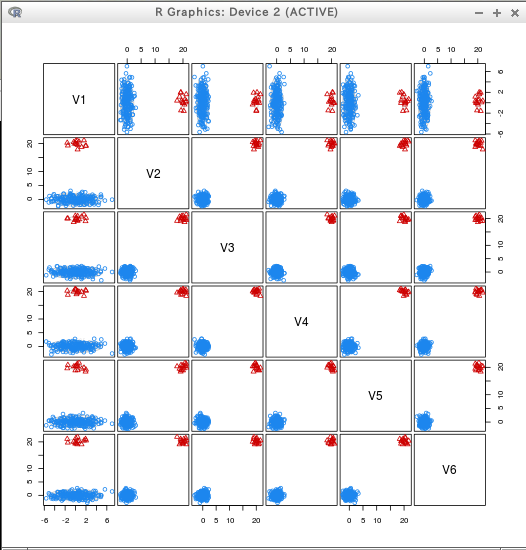
* iris
require(mclust)
C3 = Mclust(iris[,c(1:4)])
dens = densityMclust(iris[,c(1:4)])
plot(dens, iris[,c(1:4)], col = "grey",
points.col = mclust.options()$classPlotColors[C3$classification],
pch = C3$classification)
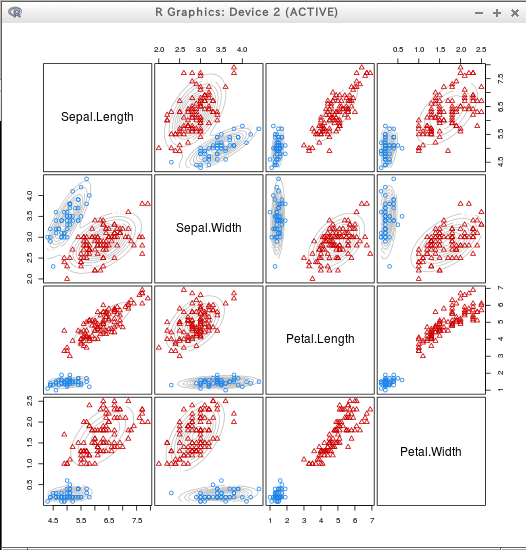
prediction
require(mclust)
C3 = Mclust(iris[,c(1:4)])
ct <- table(iris$Species, C3$classification)
ct
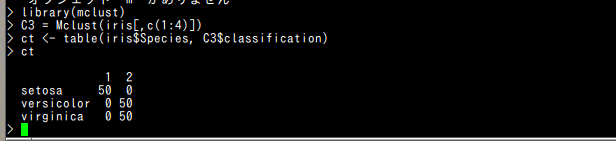